Selected Publications
MesoGraph: Automatic profiling of mesothelioma subtypes from histological images
Mark Eastwood, Heba Sailem, Silviu Marc, Sanjay Popat, Fayyaz Minhas, Jan-Lukas Robertus, 2023, Cell Reports Medicine
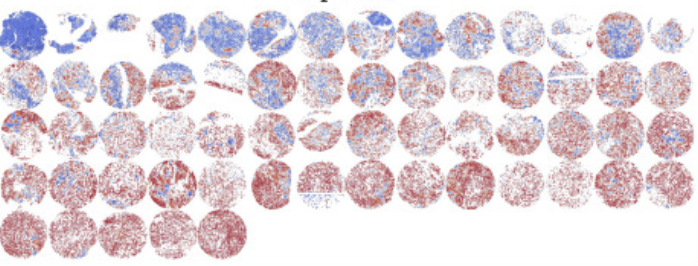
Mesothelioma is a lethal disease with survival rates of less than 10% over a five-year period. It develops in the thin layer of pleural lining and is primarily associated with asbestos exposure. A major challenge in mesothelioma diagnosis is the heterogeneity within tumours resulting in high inter-observer variability.
This research demonstrates the potential of AI in identifying distinct cellular patterns in histopathological images. By depicting a tumour as a network of interacting cells, this approach not only considers individual cell characteristics but also their surrounding microenvironment. The system's reliability was assessed through morphological analysis of predicted cell scores which confirmed consistency with epithelioid and sarcomatoid morphologies. Importantly, the output of our model can be visualized in a user-friendly interactive graphical user interface (GUI) which can be guide pathologist assessment of histopathological imaging.
[PDF]ShapoGraphy : A User-Friendly Web Application for Creating Bespoke and Intuitive Visualisation of Biomedical Data
Muhammed Khawatmi, Yoann Steux, Saddam Zourob and Heba Sailem*, 2022, Frontiers in Bioinformatics
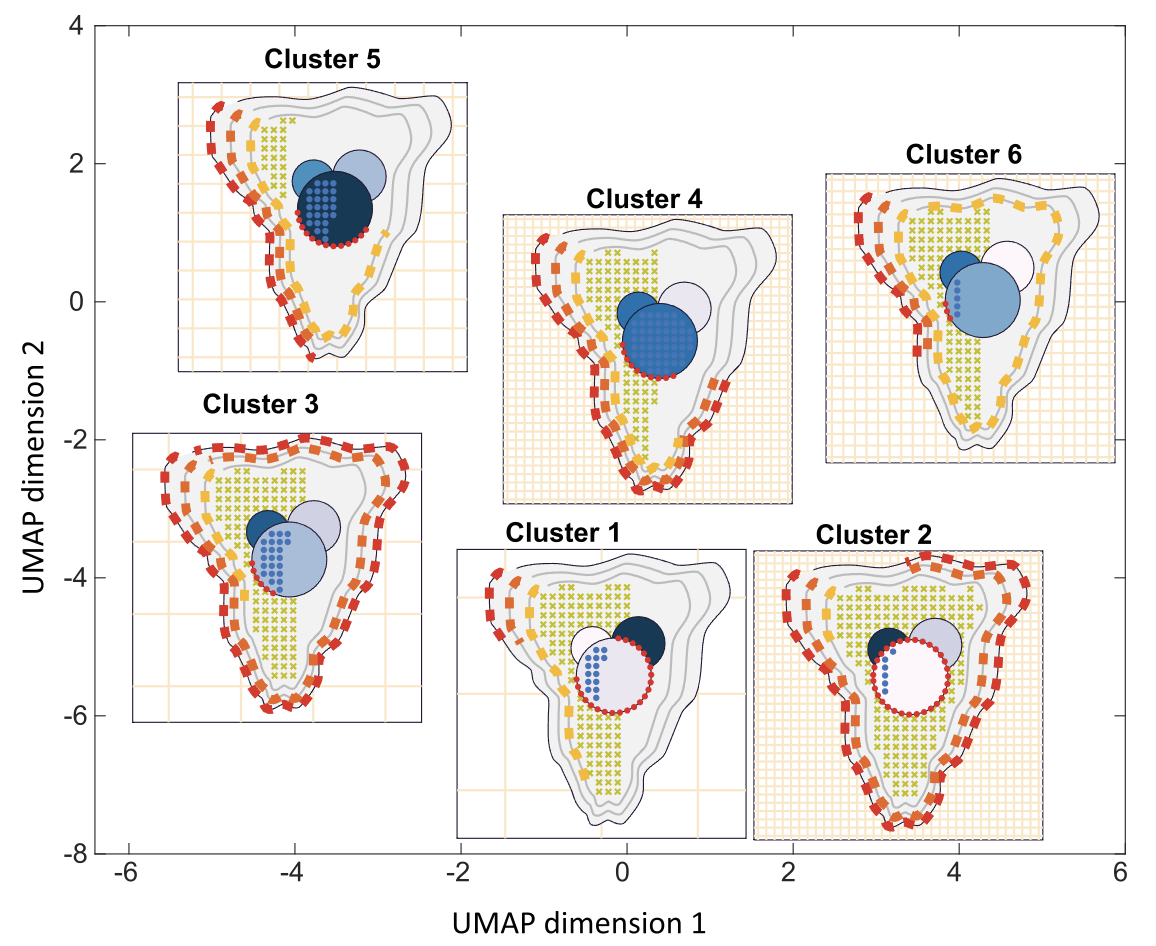
Website: www.shapography.com
Effective visualisation of quantitative microscopy data is crucial for interpreting and discovering new patterns from complex bioimage data. Existing visualisation approaches, such as bar charts, scatter plots and heat maps, do not accommodate the complexity of visual information present in microscopy data. Here we develop ShapoGraphy, a first of its kind method accompanied by an interactive web-based application for creating customisable quantitative pictorial representations to facilitate the understanding and analysis of image datasets (www.shapography.com). ShapoGraphy enables the user to create a structure of interest as a set of shapes. Each shape can encode different variables that are mapped to the shape dimensions, colours, symbols, or outline. We illustrate the utility of ShapoGraphy using various image data, including high dimensional multiplexed data. Our results show that ShapoGraphy allows a better understanding of cellular phenotypes and relationships between variables. In conclusion, ShapoGraphy supports scientific discovery and communication by providing a rich vocabulary to create engaging and intuitive representations of diverse data types. [PDF]
KCML: a machine-learning framework for inference of multi-scale gene functions from genetic perturbation screens
Heba Sailem*, Jens Rittscher, and Lucas Pelkmans, 2020, Molecular Systems Biology Winner of Early Career Bioinformatician Award 2021
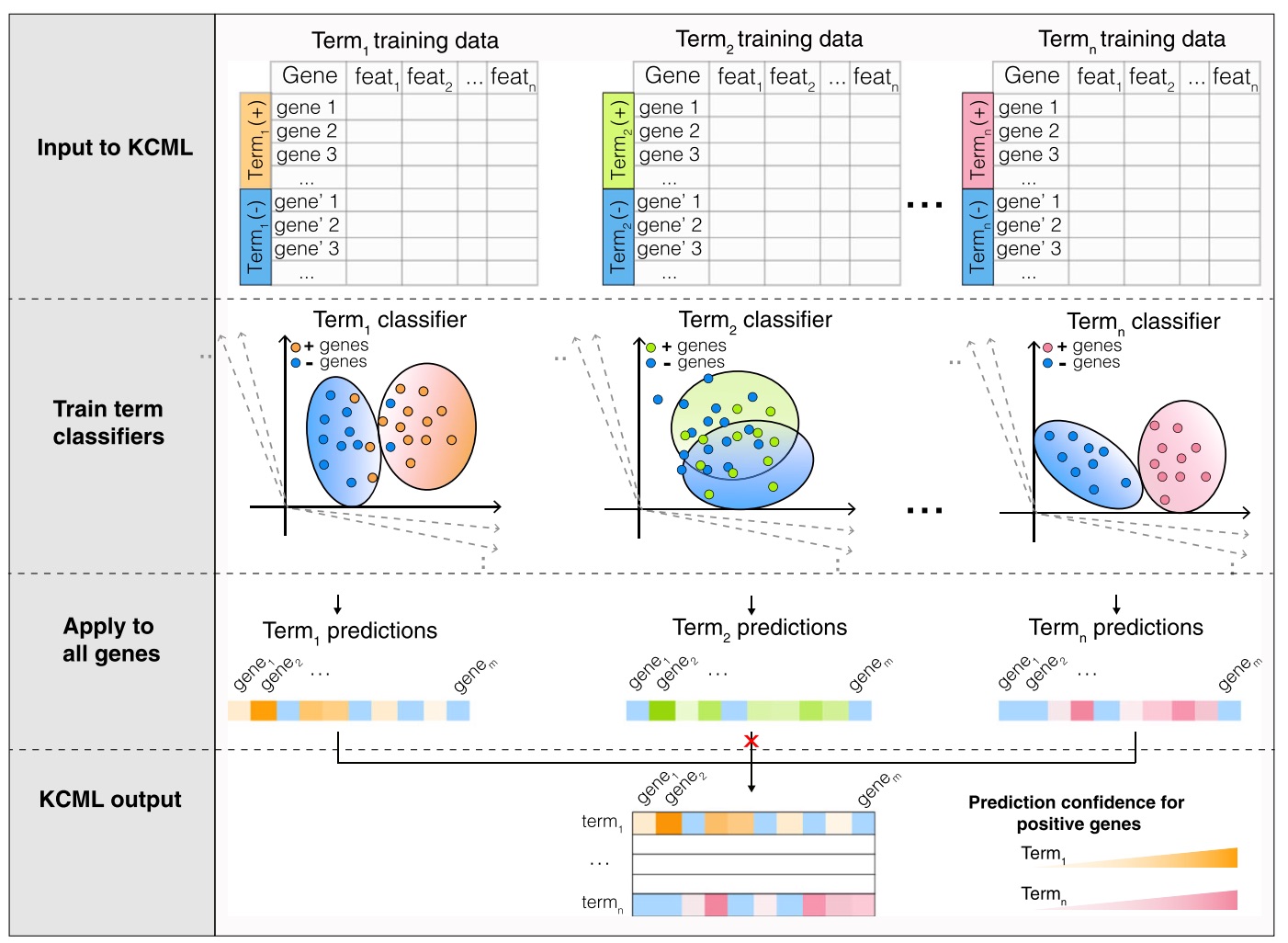
Characterising context-dependent gene functions is crucial for understanding the genetic bases of health and disease. To date, inference of gene functions from large-scale genetic perturbation screens is based on ad hoc analysis pipelines involving unsuper- vised clustering and functional enrichment. We present Knowl- edge- and Context-driven Machine Learning (KCML), a framework that systematically predicts multiple context-specific functions for a given gene based on the similarity of its perturbation phenotype to those with known function. As a proof of concept, we test KCML on three datasets describing phenotypes at the molecular, cellular and population levels and show that it outperforms traditional analysis pipelines. In particular, KCML identified an abnormal multicellular organisation phenotype associated with the depletion of olfactory receptors, and TGFb and WNT signalling genes in colorectal cancer cells. We validate these predictions in colorectal cancer patients and show that olfactory receptors expression is predictive of worse patient outcomes. These results highlight KCML as a systematic framework for discovering novel scale-crossing and context-dependent gene functions. KCML is highly generalis- able and applicable to various large-scale genetic perturbation screens. [PDF]
DeepScratch: Single-cell based topological metrics of scratch wound assays
Avelino Javer, Jens Rittscher and Heba Sailem*, 2020, Computational and Structural Biotechnology Journal
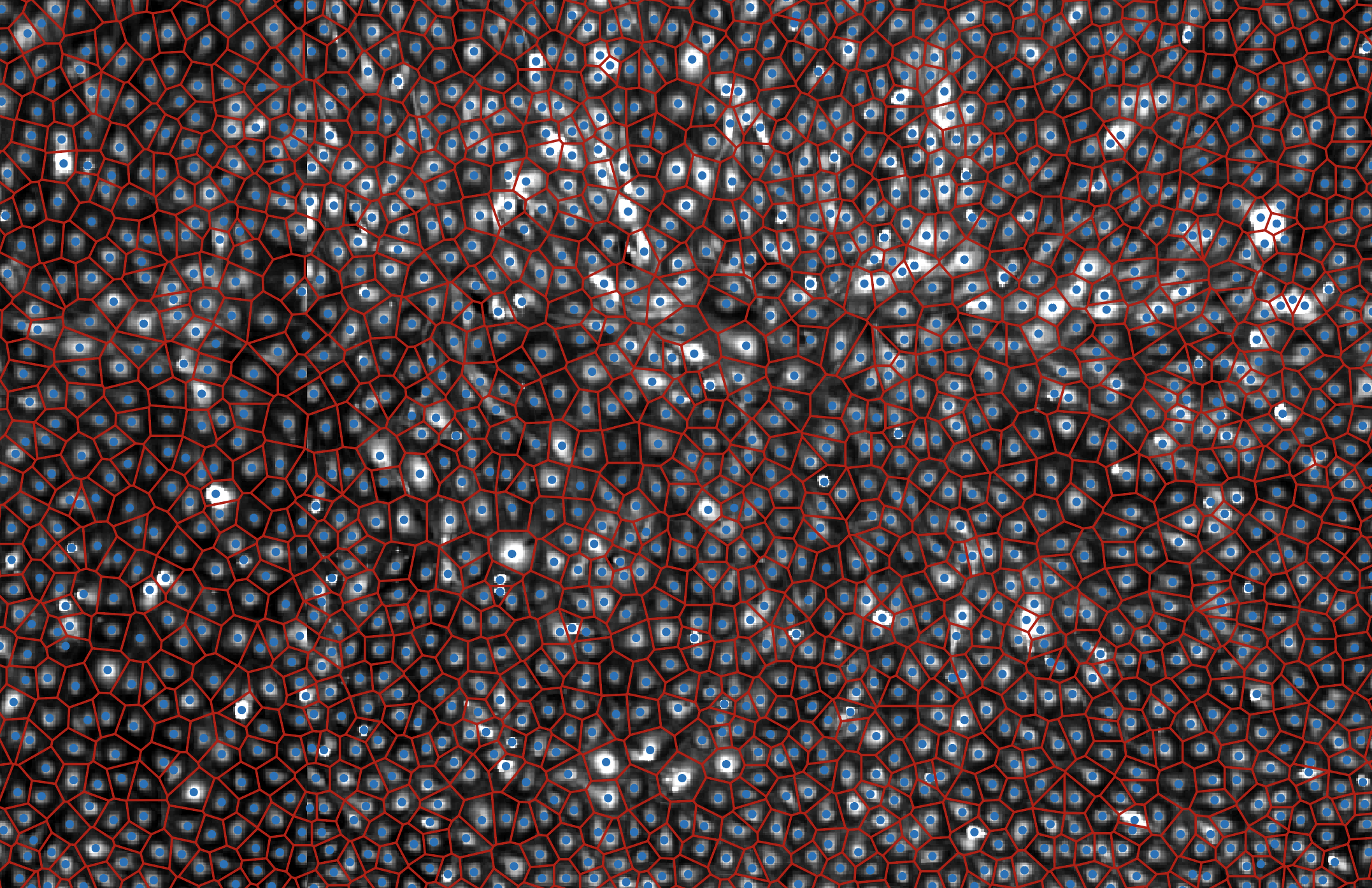
Changes in tissue architecture and multicellular organisation contribute to many diseases, including cancer and cardiovascular diseases. Scratch wound assay is a commonly used tool that assesses cells’ migratory ability based on the area of a wound they cover over a certain time. However, analysis of changes in the organisational patterns formed by migrating cells following genetic or pharmacological perturbations are not well explored in these assays, in part because analysing the resulting imaging data is challenging. Here we present DeepScratch, a neural network that accurately detects the cells in scratch assays based on a heterogeneous set of markers. We demonstrate the utility of DeepScratch by analysing images of more than 232,000 lymphatic endothelial cells. In addition, we propose various topological measures of cell connectivity and local cell density (LCD) to characterise tissue remodelling during wound healing. We show that LCD-based metrics allow classification of CDH5 and CDC42 genetic perturbations that are known to affect cell migration through different biological mechanisms. Such differences cannot be captured when considering only the wound area. Taken together, single-cell detection using DeepScratch allows more detailed investigation of the roles of various genetic components in tissue topology and the biological mechanisms underlying their effects on collective cell migration. [PDF]
Morphological landscape of endothelial cell networks reveals a functional role of glutamate receptors in angiogenesis
Heba Sailem* and Ayaman Al-Haj-Zen, 2020, Scientific Reports
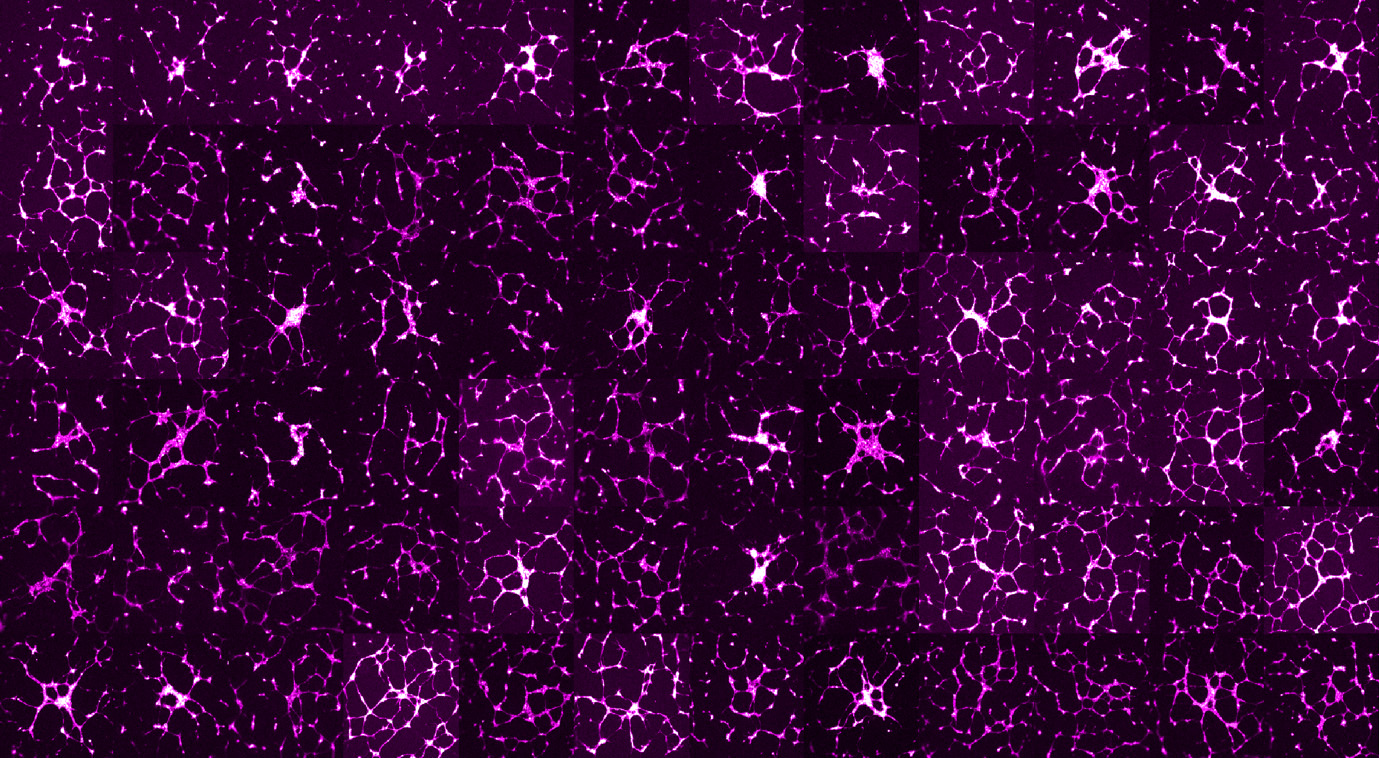
Angiogenesis plays a key role in several diseases including cancer, ischemic vascular disease, and Alzheimer’s disease. chemical genetic screening of endothelial tube formation provides a robust approach for identifying signalling components that impact microvascular network morphology as well as endothelial cell biology. However, the analysis of the resulting imaging datasets has been limited to a few phenotypic features such as the total tube length or the number of branching points. Here we developed a high content analysis framework for detailed quantification of various aspects of network morphology including network complexity, symmetry and topology. By applying our approach to a high content screen of 1,280 characterised drugs, we found that drugs that result in a similar phenotype share the same mechanism of action or common downstream signalling pathways. our multiparametric analysis revealed that a group of glutamate receptor antagonists enhances branching and network connectivity. Using an integrative meta-analysis approach, we validated the link between these receptors and angiogenesis. We further found that the expression of these genes is associated with the prognosis of Alzheimer’s patients. In conclusion, our work shows that detailed image analysis of complex endothelial phenotypes can reveal new insights into biological mechanisms modulating the morphogenesis of endothelial networks and identify potential therapeutics for angiogenesis-related diseases. [PDF]
DeepSplit: Segmentation of Microscopy Images Using Multi-Task Convolutional Networks
Andrew Torr, Doga Basaran, Julia Sero, Jens Rittscher and Heba Sailem*, 2020, In Medical Image Understanding and Analysis
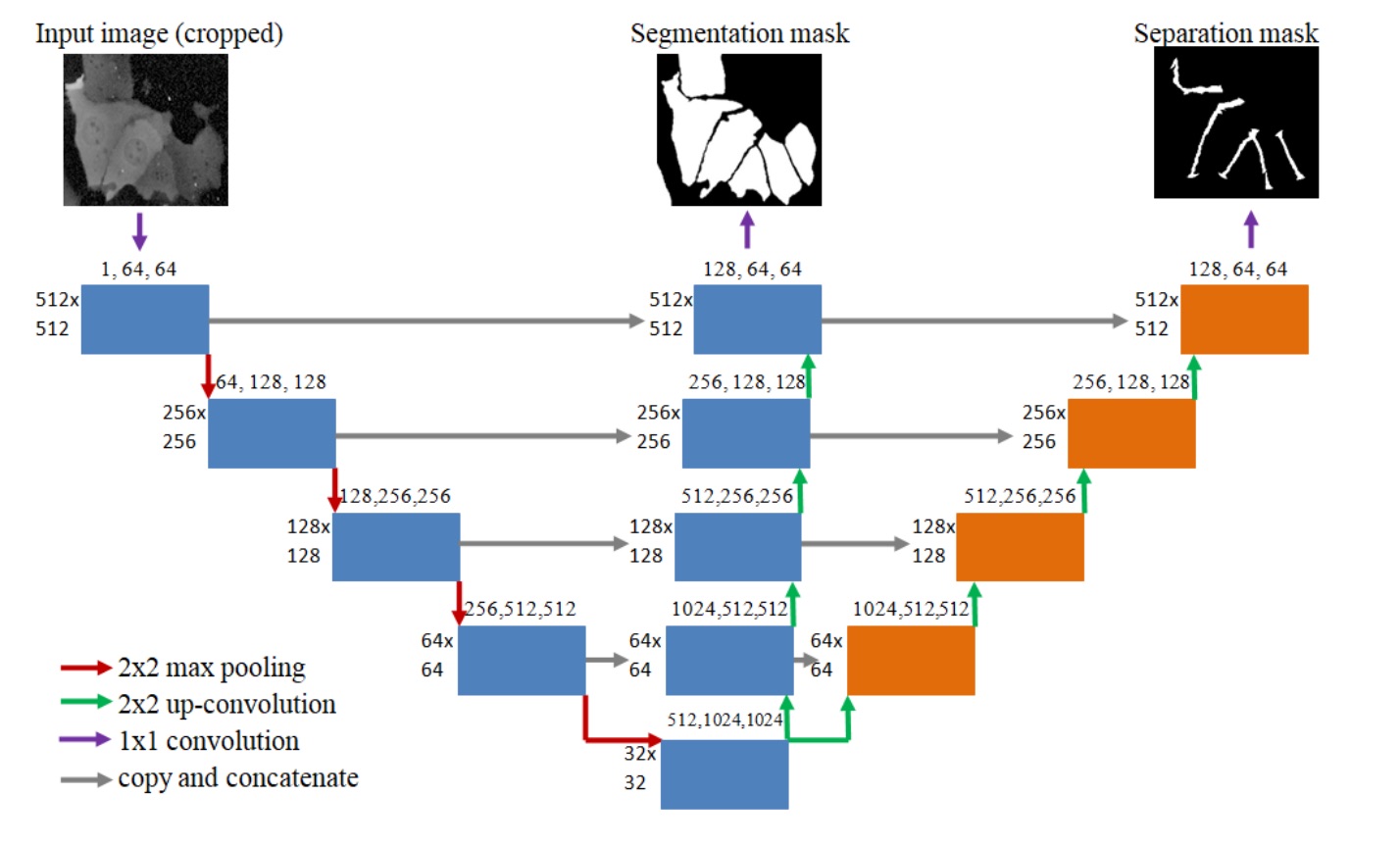
A bespoke U-NET architecture that aim at tackling the challenge of undersegmentation by explicity learning to split neighbouring cells. [PDF]
Identification of clinically predictive metagenes that encode components of a network coupling cell shape to transcription by image-omics
Heba Sailem* and Chris Bakal, 2017, Genome Research
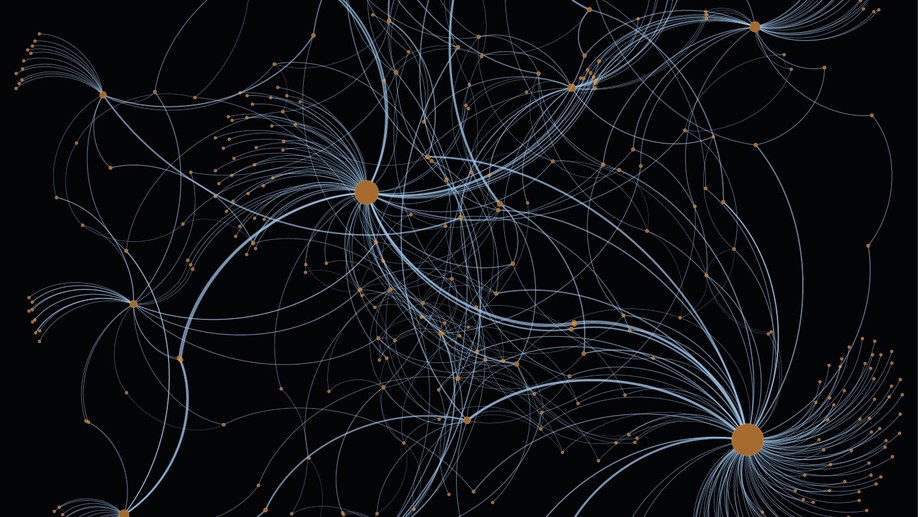
Development of an image-omic pipeline for inference of signaling networks linking the shape of breast cells to their transcriptional activities. Through this pipeline we identified genes that are predictive of the outcome of breast cancer patients. [PDF]
Visualizing cellular imaging data using PhenoPlot
Heba Sailem*, Julia Sero, and Chris Bakal, 2015, Nature Communication
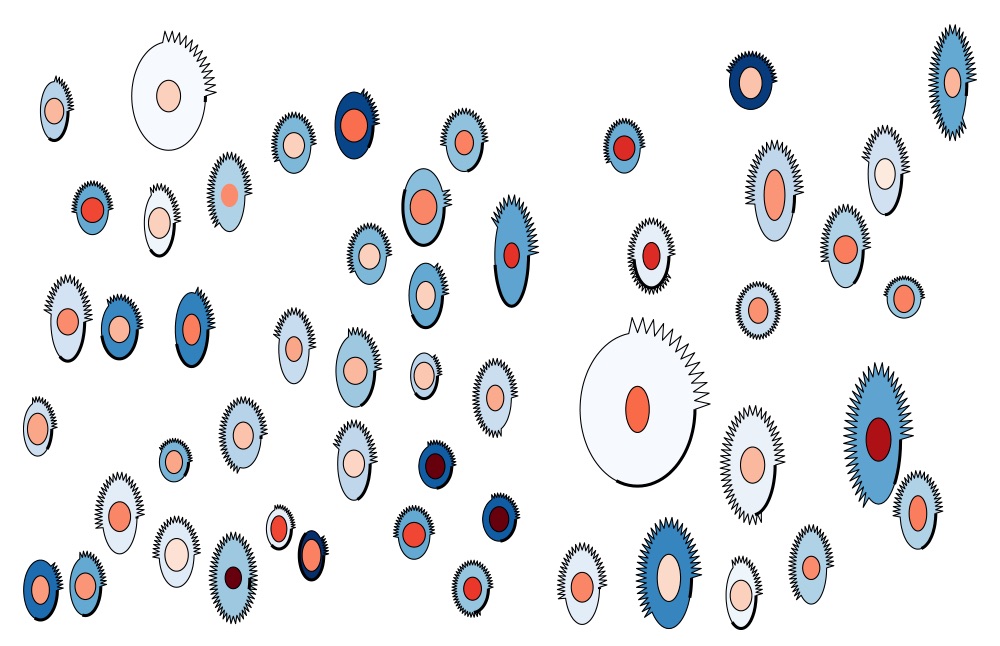
Visualization is essential for data interpretation, hypothesis formulation and communication of results. However, there is a paucity of visualization methods for image-derived data sets generated by high-content analysis in which complex cellular phenotypes are described as high-dimensional vectors of features. Here we present a visualization tool, PhenoPlot, which represents quantitative high-content imaging data as easily interpretable glyphs, and we illustrate how PhenoPlot can be used to improve the exploration and interpretation of complex breast cancer cell phenotypes. [PDF]
Discovery of Rare Phenotypes in Cellular Images Using Weakly Supervised Deep Learning
Heba Sailem, Andrew Zisserman, and Jens Rittscher, 2017, In International Conference of Computer Vision workshop
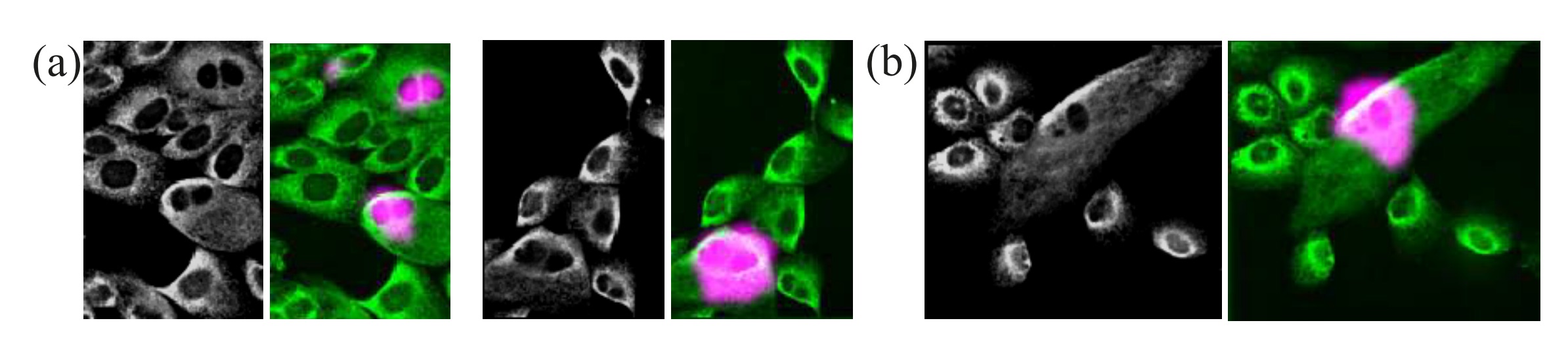
High-throughput microscopy generates a massive amount of images that enables the identification of bio- logical phenotypes resulting from thousands of different genetic or pharmacological perturbations. However, the size of the data sets generated by these studies makes it almost impossible to provide detailed image annotations, e.g. by object bounding box. Furthermore, the variability in cellular responses often results in weak phenotypes that only manifest in a subpopulation of cells. To overcome the burden of providing object-level annotations we propose a deep learning approach that can detect the presence or absence ofrare cellular phenotypes from weak annotations. Although, no localization information is provided we demonstrate that our Weakly Supervised Convolutional Neural Network (WSCNN) can reliably estimate the location of the identified rare events. Results on synthetic data set and a data set containing genetically perturbed cells demonstrate the power ofour proposed approach. [PDF]
Microenvironmental Heterogeneity Parallels Breast Cancer Progression: A Histology– Genomic Integration Analysis
Rachael Natrajan, Heba Sailem, Faraz K. Mardakheh, Mar Arias Garcia, Christopher J. Tape, Mitch Dowsett, Chris Bakal and Yinyin Yuan, 2016, PLOS Medicine
Background: The intra-tumor diversity of cancer cells is under intense investigation; however, little is known about the heterogeneity of the tumor microenvironment that is key to cancer progres- sion and evolution. We aimed to assess the degree of microenvironmental heterogeneity in breast cancer and correlate this with genomic and clinical parameters. Methods: and Findings We developed a quantitative measure of microenvironmental heterogeneity along three spatial dimensions (3-D) in solid tumors, termed the tumor ecosystem diversity index (EDI), using fully automated histology image analysis coupled with statistical measures commonly used in ecology. This measure was compared with disease-specific survival, key mutations, genome-wide copy number, and expression profiling data in a retrospective study of 510 breast cancer patients as a test set and 516 breast cancer patients as an independent vali- dation set. In high-grade (grade 3) breast cancers, we uncovered a striking link between high microenvironmental heterogeneity measured by EDI and a poor prognosis that cannot be explained by tumor size, genomics, or any other data types. However, this association was not observed in low-grade (grade 1 and 2) breast cancers. The prognostic value of EDI was superior to known prognostic factors and was enhanced with the addition of TP53 mutation status (multivariate analysis test set, p =9× 10−4, hazard ratio = 1.47, 95% CI 1.17–1.84; validation set, p = 0.0011, hazard ratio = 1.78, 95% CI 1.26–2.52). Integration with genome-wide profiling data identified losses of specific genes on 4p14 and 5q13 that were enriched in grade 3 tumors with high microenvironmental diversity that also substrati- fied patients into poor prognostic groups. Limitations of this study include the number of celltypes included in the model, that EDI has prognostic value only in grade 3 tumors, and that our spatial heterogeneity measure was dependent on spatial scale and tumor size. Conclusions: To our knowledge, this is the first study to couple unbiased measures of microenvironmental heterogeneity with genomic alterations to predict breast cancer clinical outcome. We pro- pose a clinically relevant role of microenvironmental heterogeneity for advanced breast tumors, and highlight that ecological statistics can be translated into medical advances for identifying a new type of biomarker and, furthermore, for understanding the synergistic inter- play of microenvironmental heterogeneity with genomic alterations in cancer cells.
Cell shape and the microenvironment regulate nuclear translocation of NF-kB in breast epithelial and tumor cells
Julia Sero*, Heba Sailem*, Rico Chandra Ardy, Hannah Almuttaqi, Tongli Zhang and Chris Bakal, 2015, Molecular Systems Biology
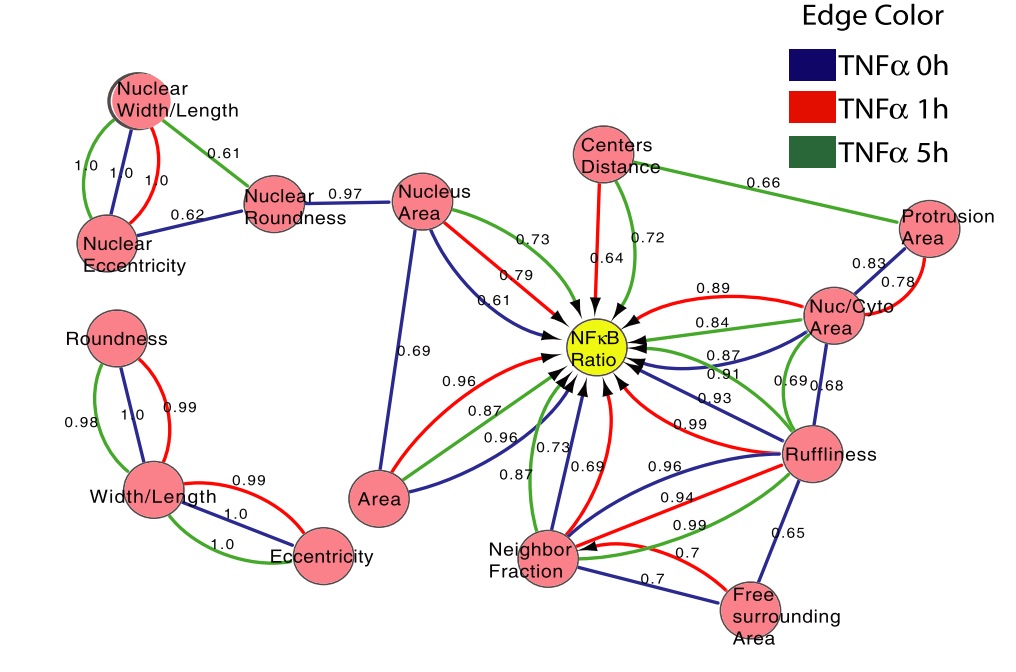
Although a great deal is known about the signaling events that promote nuclear translocation of NF-kappaB, how cellular biophysics and the microenvironment might regulate the dynamics of this pathway is poorly understood. In this study, we used high-content image analysis and Bayesian network modeling to ask whether cell shape and context features influence NF-kappaB activation using the inherent variability present in unperturbed populations of breast tumor and non-tumor cell lines. Cell–cell contact, cell and nuclear area, and protrusiveness all contributed to variability in NF-jB localization in the absence and presence of TNFa. Higher levels of nuclear NF-kappaB were associated with mesenchymal-like versus epithelial-like morphologies, and RhoA-ROCK-myosin II signaling was critical for mediating shape-based differences in NF-kappaB localization and oscillations. Thus, mechanical factors such as cell shape and the microenvironment can influence NF-kappaB signaling and may in part explain how different phenotypic outcomes can arise from the same chemical cues. [PDF]
A screen for morphological complexity identifies regulators of switch-like transitions between discrete cell shapes
Zheng Yin, Amine Sadok, Heba Sailem, ..., Stephen T. C. Wong, Chris Bakal, 2013, Nature Cell Biology
The way in which cells adopt different morphologies is not fully understood. Cell shape could be a continuous variable or restricted to a set of discrete forms. We developed quantitative methods to describe cell shape and show that Drosophila haemocytes in culture are a heterogeneous mixture of five discrete morphologies. In an RNAi screen of genes affecting the morphological complexity of heterogeneous cell populations, we found that most genes regulate the transition between discrete shapes rather than generating new morphologies. In particular, we identified a subset of genes, including the tumour suppressor PTEN, that decrease the heterogeneity of the population, leading to populations enriched in rounded or elongated forms. We show that these genes have a highly conserved function as regulators of cell shape in both mouse and human metastatic melanoma cells. [PDF]